In her July 16 seminar, Christina Ramirez, a professor of Biostatistics at the UCLA Fielding School of Public Health, shared her groundbreaking, comprehensive model that combined traditional SEIR models with case velocity and machine learning to get precise, reliable estimates of COVID-19 case and death rates — shining a light on whether the pandemic is gaining speed and if deaths are accelerating or stabilizing. This project also uses the UCLA Center for Health Policy Research’s California Health Interview Survey (CHIS) to obtain an accurate snapshot of California data so that morbidity and mortality rates are based on the known prevalence of sociodemographic factors such as age, race, and co-morbidities or underlying health conditions.
Event Navigation
Combining Traditional Modeling with Machine Learning for Predicting COVID-19
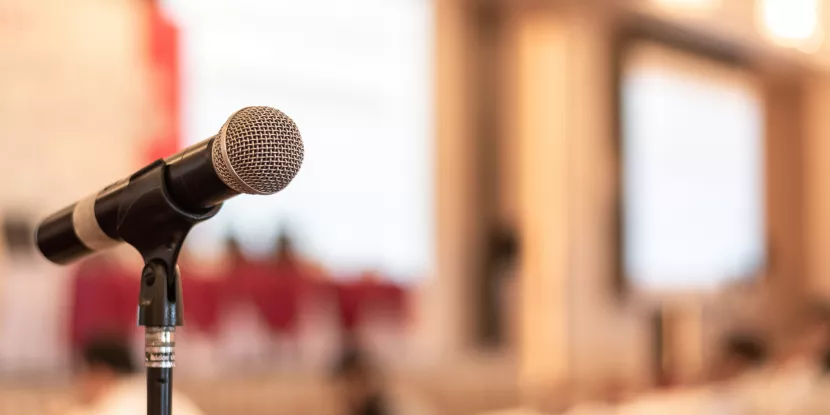
Online
Combining Traditional Modeling with Machine Learning for Predicting COVID-19